Have you ever thought that it would have been great if you didn’t have to select Genre in your music app manually and rather your app should recognize the genre of the song through itself just by listening to the song or customize your song library according to the genre of the song that you listen most.
Well in today’s world with the power of Deep Learning everything is possible, you could build a deep learning model which can learn to distinguish songs on the basis of their genre by looking at their audio features.
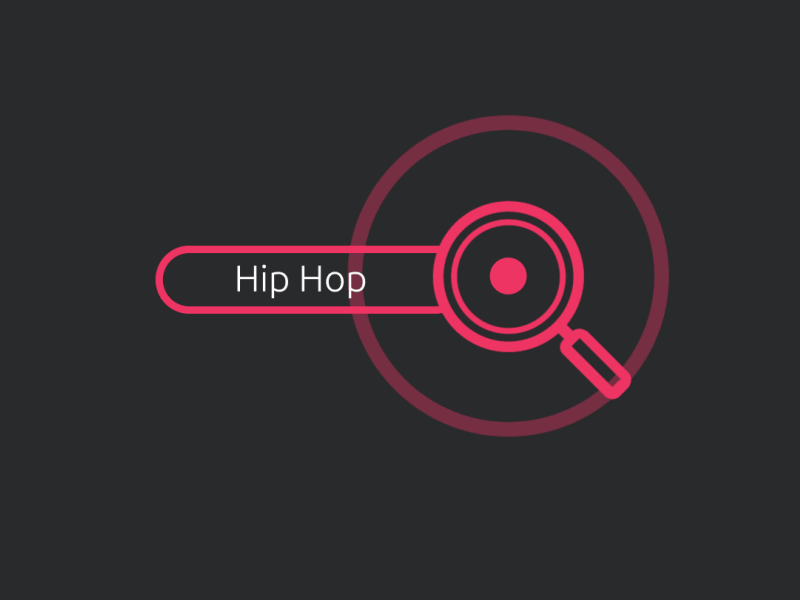
Introduction to cAInvas
cAInvas is an integrated development platform. Not only we can train our deep learning model using Tensorflow, Keras, or Pytorch we can also compile our model with its edge compiler called DeepC to deploy our working model on edge devices for production. The Song Genre Prediction model which we are going to talk about was developed in cAInvas.
Why cAInvas
cAInvas is an ideal choice to develop Deep Learning models because of the simplicity it provides to develop even the most complex Deep Learning Models and also because of an amazing UseCase Gallery which contains pre-developed notebooks for the development of various Deep Learning Models.
Going through them will help you gain intuition about how Deep Learning Models are developed. It also provides GPU support for training Deep Learning Models. Song Genre Prediction is also part of the cAInvas’ gallery.
Source of Data
While working in cAInvas one of its key features is UseCases Gallary. When working on any of its UseCases you don’t have to look for data manually. As they have the feature to import your dataset to your workspace when you work on them. To load the data we just have to enter the following commands:
Data Analysis
The dataset provided is already pre-processed and the various audio features like Chroma Shift, RMSE, MFCC, Spectral Centroid, Spectral Bandwidth, and Zero Crossing Rate has already been extracted from the audio files and have been stored along with their labels in a .csv file.
So, this will save most of the time as we don’t have to extract the audio features by ourselves for training the Deep Learning Models. All together total of twenty-six different features have been extracted and stored in the dataset. The songs provided in the dataset belongs to ten different genre.
Data Preprocessing and Test-Train Split
The dataset has been loaded via Pandas in the form of dataframe and since all the features are important for model training, we won’t be dropping any feature column from our dataset but we will scale all the features in our dataset by using Scikit Learn’s Standard Scalar Module and we will one-hot encode our labels for the training purpose of our model.
We will also do the test-train split by keeping the 75% of the dataset for training the model and the rest 25% of the dataset for model evaluation. All this can be done by executing the following commands:
Model Training
After Data preprocessing and Creation of TestSet and TrainSet, next step is to pass our extracted them for our Deep Learning model along with the target labels so that our model can learn to classify song genre.
The model architecture used was:
Model: "sequential" _________________________________________________________________ Layer (type) Output Shape Param # ================================================================= dense (Dense) (None, 512) 13824 _________________________________________________________________ dropout (Dropout) (None, 512) 0 _________________________________________________________________ dense_1 (Dense) (None, 256) 131328 _________________________________________________________________ dropout_1 (Dropout) (None, 256) 0 _________________________________________________________________ dense_2 (Dense) (None, 64) 16448 _________________________________________________________________ dropout_2 (Dropout) (None, 64) 0 _________________________________________________________________ dense_3 (Dense) (None, 10) 650 ================================================================= Total params: 162,250 Trainable params: 162,250 Non-trainable params: 0 _________________________________________________________________
The loss function used was “categorical_crossentropy” and optimizer used was “Adam”.For training the model we used Keras API with tensorflow at backend. The model achieved a decent accuracy and showed a good performance.
Here are the training plots for the model:
Accessing the Model’s Performance
Next step was to access the performance of the model so as to see how well does it classify songs on the basis of their genre. For this we used the TestSet and passed it to our model to obtain the predictions. We displayed the first ten predictions along with the actual labels. And we observed that model is doing well to detect the song genre.
Introduction to DeepC
DeepC Compiler and inference framework is designed to enable and perform deep learning neural networks by focussing on features of small form-factor devices like micro-controllers, eFPGAs, cpus and other embedded devices like raspberry-pi, odroid, arduino, SparkFun Edge, risc-V, mobile phones, x86 and arm laptops among others.
DeepC also offers ahead of time compiler producing optimized executable based on LLVM compiler tool chain specialized for deep neural networks with ONNX as front end.
Compilation with DeepC
After training the model, it was saved in an H5 format using Keras as it easily stores the weights and model configuration in a single file. For this we used Keras’ Modelcheckpoint and saved the best model while training the model.
After saving the file in H5 format we can easily compile our model using DeepC compiler which comes as a part of cAInvas platform so that it converts our saved model to a format which can be easily deployed to edge devices. And all this can be done very easily using a simple command.
And our model is ready for deployment on edge devices.👍
Link for the cAInvas Notebook: https://cainvas.ai-tech.systems/use-cases/song-genre-prediction-app/
Credit: Ashish Arya
Also Read: Hit Song Prediction